Image Quality Assessment Crack Download X64
- tigfevertimispo
- May 19, 2022
- 5 min read
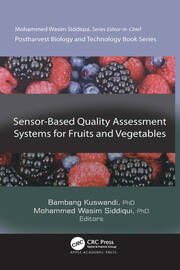
Image Quality Assessment Crack Free In general, IQA methods measure the similarity between two images that have been encoded using different techniques. This is done by correlating a reference image (one which has already been encoded) to a distorted or degraded version of the same image (the one that will be encoded). This comparison is a way to determine how distortion, or degradation, affects the perceptual quality of an image, more specifically, how much it compromises the overall quality. The three main categories of IQA methods can be grouped into three types: · Structural-based methods The first category, called structural based methods, which can be further split into: · Group invariant methods · Patch-based methods . Group invariant methods are much faster and use the similarity between the relevant part of the image, called group invariant descriptor (GID) in order to obtain a quality score. One of the best and fastest group invariant descriptors is the GIST descriptor that is fast to extract and easy to compare for different images. . Patch-based methods attempt to find image distortions on local patches which can be encoded in GID in order to obtain quality scores. They do it in a hierarchical fashion where each block of the image is considered as a patch and the quality of each block is given as a weighted average of the quality scores of the patches that are inside it. The weighting of each block is a function of how much the block is distorted and how much the distortion is relevant. . This family of methods is considered as the most accurate ones as the visual effects of distortions on different parts of the image are taken into account. · Model-based methods . Model-based methods are the second category which have been expanded to adapt to changes in the media over time. The image quality metrics in this category are more complex in order to account for the best distortion factors of any image. They are slower than the previous ones. This category can be further split into: . Quantization based methods . Dithering based methods . Quantization based methods use the statistics of the quantization process in order to find the best quantization parameters. These parameters are the best trade-off between the quantization error and the number of bits that are required to encode the image. . Dithering based methods attempt to achieve the best quantization error while keeping the number of quantization bits low. They require the use of dithering matrices in order to add noise to the image prior to quantization in order to get a better Image Quality Assessment Crack+ With Full Keygen [Latest 2022] The library also implements the SCI JPEG 2000 algorithm but it is not supported by the official SCI JPEG 2000 toolkit. - Why does the PSNR metric support a quality value for the MSE? The PSNR metric was born to evaluate the MSE metric in the case of JPEG images. In the case of JPEG images the MSE metric is not constant in low bitrate. Indeed, it decreases as the number of samples increases. To evaluate an image using the PSNR metric, the worst value of the MSE should be used. In this case we have to use the MSE value of the worst frame in order to evaluate the image. - How to use the PSNR metric? The PSNR metric requires an additional parameter called quality. This value represents the maximum quality of the image. This parameter is optional. If it is not specified it uses the default quality value: 50. This parameter can be useful if the quality of a single frame is very high. Example: PSNR_C=PSNR_C(x,q,q,false,false); int q=50; In this case the PSNR metric returns the PSNR metric of the frames where the quality is greater than or equal to 50. Example: PSNR_C=PSNR_C(x,q,q,false,true); int q=50; In this case the PSNR metric returns the PSNR metric of the worst frame where the quality is greater than or equal to 50. - Can the PSNR metric be used for other image formats (e.g. bmp) than JPEG? Yes, the PSNR metric can be used for all formats that support the MSE metric. However, the PSNR metric calculates the PSNR metric for the single frame. For instance, if you want to use the PSNR metric for all images in a folder you can use the following function: PSNR_C=PSNR_C(folder); Example: PSNR_C=PSNR_C(get_image(i, q)); - Why the PSNR metric supports a threshold for the detection of edge loss? The PSNR metric is always calculated on the constant region where it is possible to detect the effect of the loss of edges. In order to remove this effect, the constant region is replaced by a region defined by a threshold. Example: uint8_t* first_img_const=first_image[0]; uint8_t* last_img_const=last_image[0]; uint8_t* 1a423ce670 Image Quality Assessment (April-2022) This is a DLL that uses the low-level functions that the DirectShow.dll module has. The video is a high-definition, 10-bit resolution, interlaced video encoded with MS-MPEG-4 and it is encoded in 1080 x 1920 at 30 frames per second. The audio is an MP3 audio file encoded at 44.1 kHz, 16-bit samples and with a sampling rate of 44.1 kHz. The testing scenario is a BMP image, 8-bit and resolution of 1, 2 and 4. The program should detect and display the metrics generated by the algorithms and also display a table with the final score of each comparison. The XAudio2 is the API used by the sample for the audio processing. The visual analysis of the image is performed using the DirectShow.NET COM API. It’s necessary to set a capture graph that captures the video and audio streams and a rendering graph that will display the captured images. DARK SECOND Description: This is a DLL that uses the low-level functions that the DirectShow.dll module has. The video is a high-definition, 10-bit resolution, interlaced video encoded with MS-MPEG-4 and it is encoded in 1080 x 1920 at 30 frames per second. The audio is an MP3 audio file encoded at 44.1 kHz, 16-bit samples and with a sampling rate of 44.1 kHz. The testing scenario is a BMP image, 8-bit and resolution of 1, 2 and 4. The program should detect and display the metrics generated by the algorithms and also display a table with the final score of each comparison. The XAudio2 is the API used by the sample for the audio processing. The visual analysis of the image is performed using the DirectShow.NET COM API. The CMSampleBufferGetNumSamples() function is used to get the number of samples of the audio " [[Description]] The function inputs the CMSampleBufferRef samples buffer, then uses the CMSampleBufferGetSampleDescription() function to return the samples information in the audio buffer. The CMSampleBufferRef samples buffer must be previously created by calling the CMSampleBufferCreate() function. " [[Description]] The function inputs the CMSampleBufferRef samples buffer, then uses the CMSampleBufferGetSampleTime() function to get the samples time. " What's New in the? System Requirements: - Windows 7/Vista/XP (32-bit) or later - 2GB (minimum) of RAM - Dual Core CPU with 2.0GHz or faster - For Windows XP: 1GB of available RAM - Sound card with ALSA (Linux support) - Latest Direct3D or OpenGL drivers for your video card Minimum System Requirements: - Mac OS X 10.5 or later - Intel Core 2 Duo CPU or equivalent - For
Related links:
Comments